AI Solutions in the Manufacturing Industry: The Rise of Intelligent Production
As was established a couple of years ago by the World Economic Forum, the 4th Industrial Revolution is the most disruptive socio-economic transformation that humanity has ever faced. It generally encompasses all of the ongoing technology-enabled changes that are affecting industries and nations around the world. Self-driving cars, connected devices, AI-enabled computer chips, and many other innovations represent this monumental shift.
Just like during the last industrial revolution, manufacturing is also at the center of some of these groundbreaking advancements. Manufacturing is naturally predisposed to change, as making things cheaper and better has always been the ultimate competitive advantage. That’s why “the next Kanban” will probably be AI in manufacturing, becoming part of what is now known as smart manufacturing. According to Accenture, industrial applications of AI have the potential to be the most disruptive due to the highly competitive landscape. And 92% of manufacturing executives agree with this prediction, confirming that they see AI as one of the biggest drivers of innovation in the space.

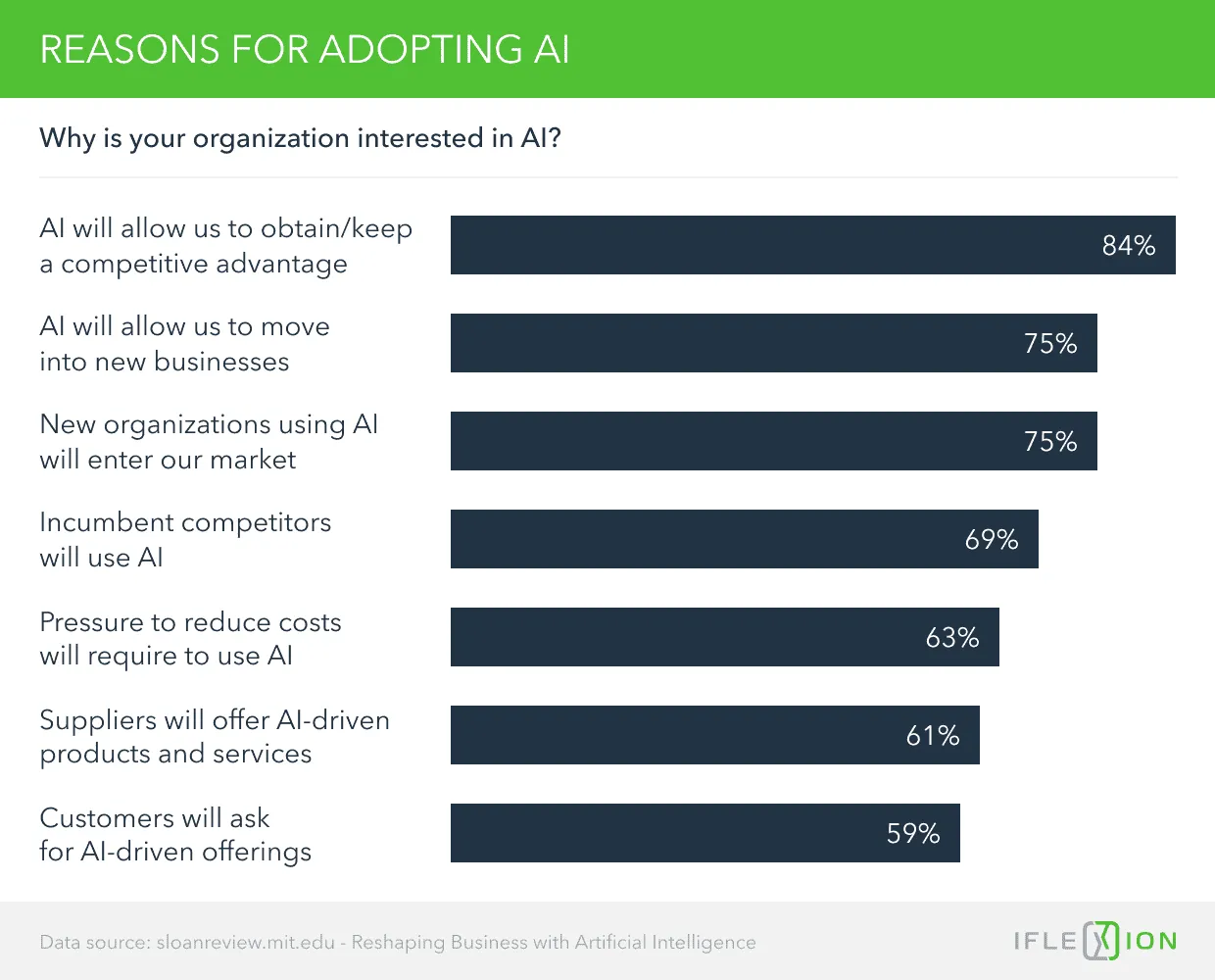
The future vector and the landscape are pretty straightforward. Innovate or perish. That’s why the artificial intelligence in manufacturing as a market is expected to grow by 50% annually according to MarketsandMarkets' report. But where do manufacturers apply these investments? And, most importantly, what are some of the most actionable applications of artificial intelligence in manufacturing?
Let’s explore the most impactful artificial intelligence use cases in manufacturing. As a reminder, we wanted to point out that AI and machine learning are often used interchangeably by us and many other sources, as in many contexts these terms mean the same thing.
R&D
Research and development are essential for manufacturing. That’s a no-brainer. Any company that innovates through R&D, like with the example of Toyota, is bound to lead the market and maybe even monopolize it.
AI in manufacturing can accelerate and improve a multitude of R&D efforts. When applied throughout the R&D process, AI can significantly accelerate product development and prototyping by:
- Identifying the optimal mix of materials for the final product
- Identifying prototypes or prototype parts that are likely to fail
- Accelerating product data exploration and discovery
These are just some example. According to Hitachi, one of the pioneers of industrial AI, the next evolutionary step will be combining automated systems powered by machine learning (AI) with rule-based systems, created with the help of expertise and human input.

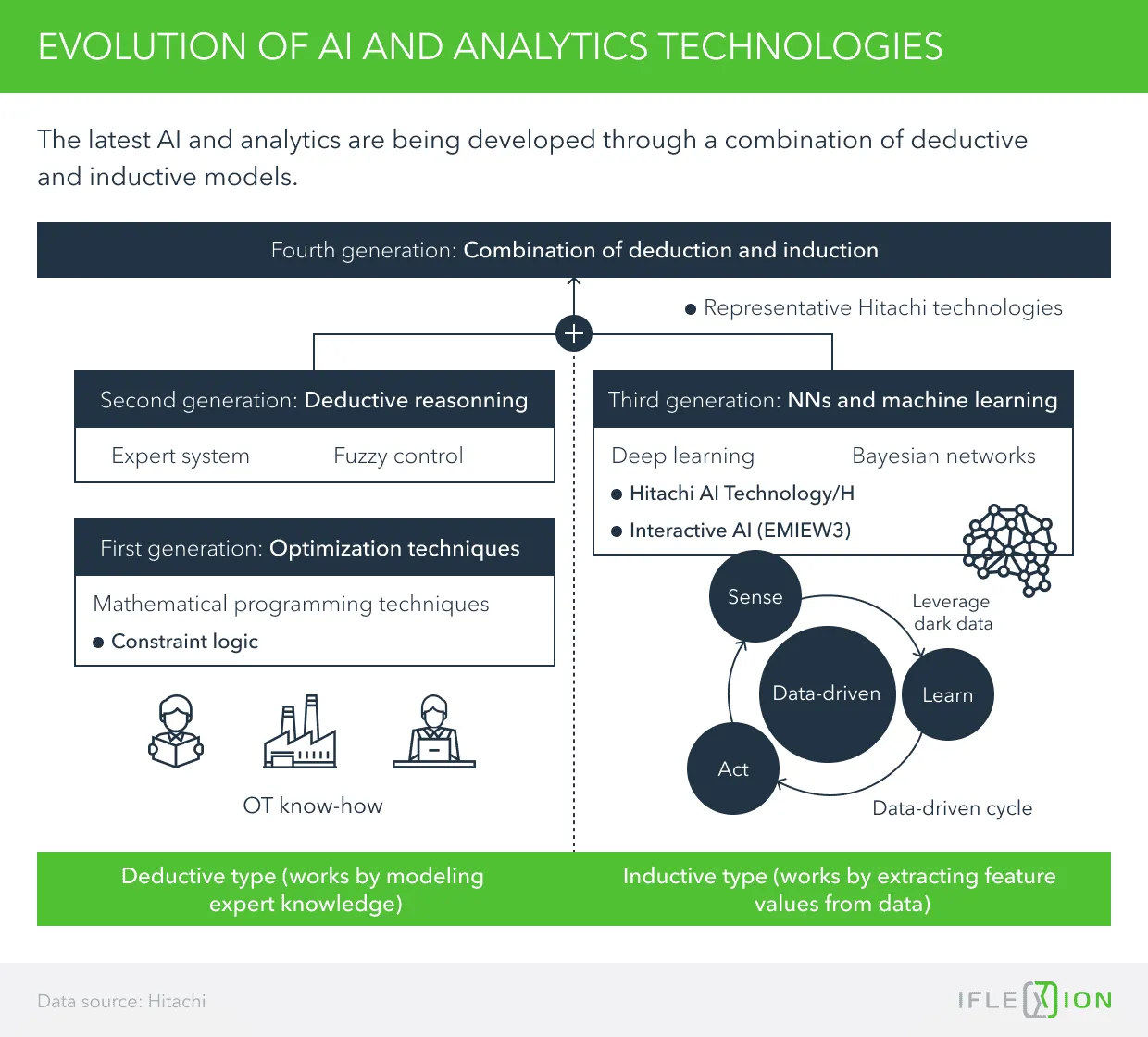
This is the reason why many companies are starting to invest in this AI niche. For example, Foxconn is investing a third of a trillion dollars over the next couple of years into AI R&D.
Production Optimization
This is a huge area of interest for manufacturers for one simple reason—cost-optimization.
The US National Institute of Standards and Technology surveyed American manufacturers. One of the top three market challenges for them was “price reduction pressure.” Among the top 10 reasons, more than half were associated with production costs.
This is where AI can make and is making a huge impact. It’s important to note that production optimization can involve a variety of very specialized niches and applications. Manufacturers can explore these as separate AI projects:
- Maintenance
- Supply Chain
- Yield Optimization
- Fault Detection / QA Automation
- Facility Operations
- Staffing
These are just some of the niches in the production environment that directly affect the cost-effectiveness of the whole manufacturing process.
Predictive Maintenance
This is one of the fastest growing niches of AI in manufacturing. Unplanned downtime already costs manufacturers billions of dollars, with a minute of downtime costing as much as $22,000. That is a staggering figure, and manufacturers are fixed on minimizing it.
The concept is pretty simple as an idea but incredibly complicated as a real-world application. You have thousands of data points being registered throughout your production line: from pressure gauges to infrared sensors and vibration detectors.
If you’re using a rule-based system, you might incorporate some of this data, but you will not be able to capture all of the correlations in their entirety, because the system is built on expert knowledge. And humans can’t physically think in that many dimensions. So you may end up with warnings or fault detection alerts when it’s too late, and the production has to be stopped.

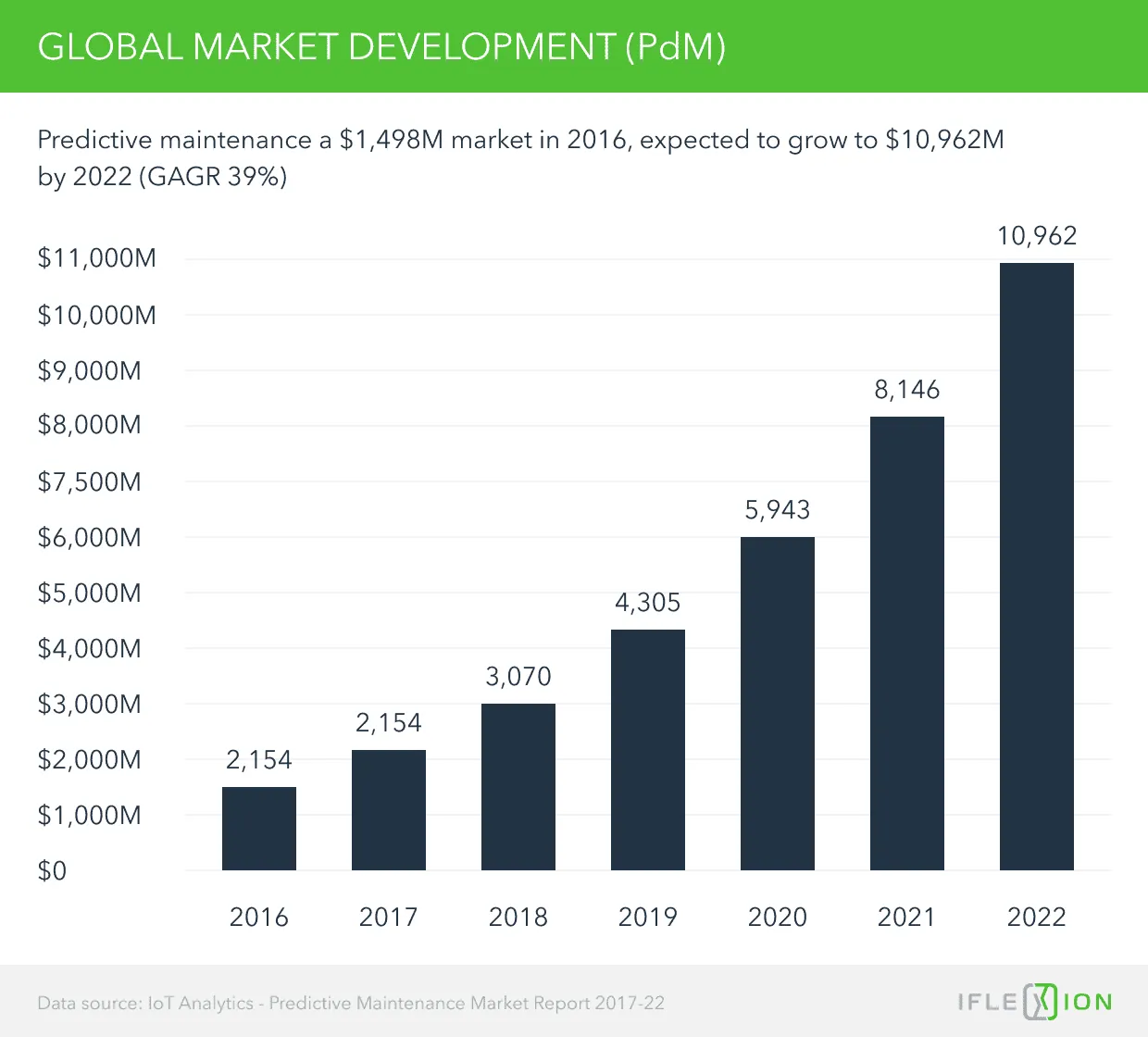
An AI system has no problem processing all of the sensor data and capturing all of the available correlations. As a result, you have an early warning system that informs you about the failure probability for each system that you monitor practically in real time. You choose which one to service first and how to optimize the schedule to decrease the downtime.
The results can be astonishing. According to Deloitte, an AI-enabled predictive maintenance system can increase equipment uptime by 10%-20%, reduce material spend by 10%, reduce maintenance planning time by up to 50%, and as a result shave off at least 10% off the overall maintenance costs. That’s millions of dollars of savings per year, with a triple-digit ROI if everything is operationalized properly.
Supply Chain Management
A complex manufacturing process can utilize tens of thousands of parts. All of them have to be sourced, delivered, and stocked. It’s essential that this chain remains highly functional and operational — it’s one of the tenants of lean manufacturing.

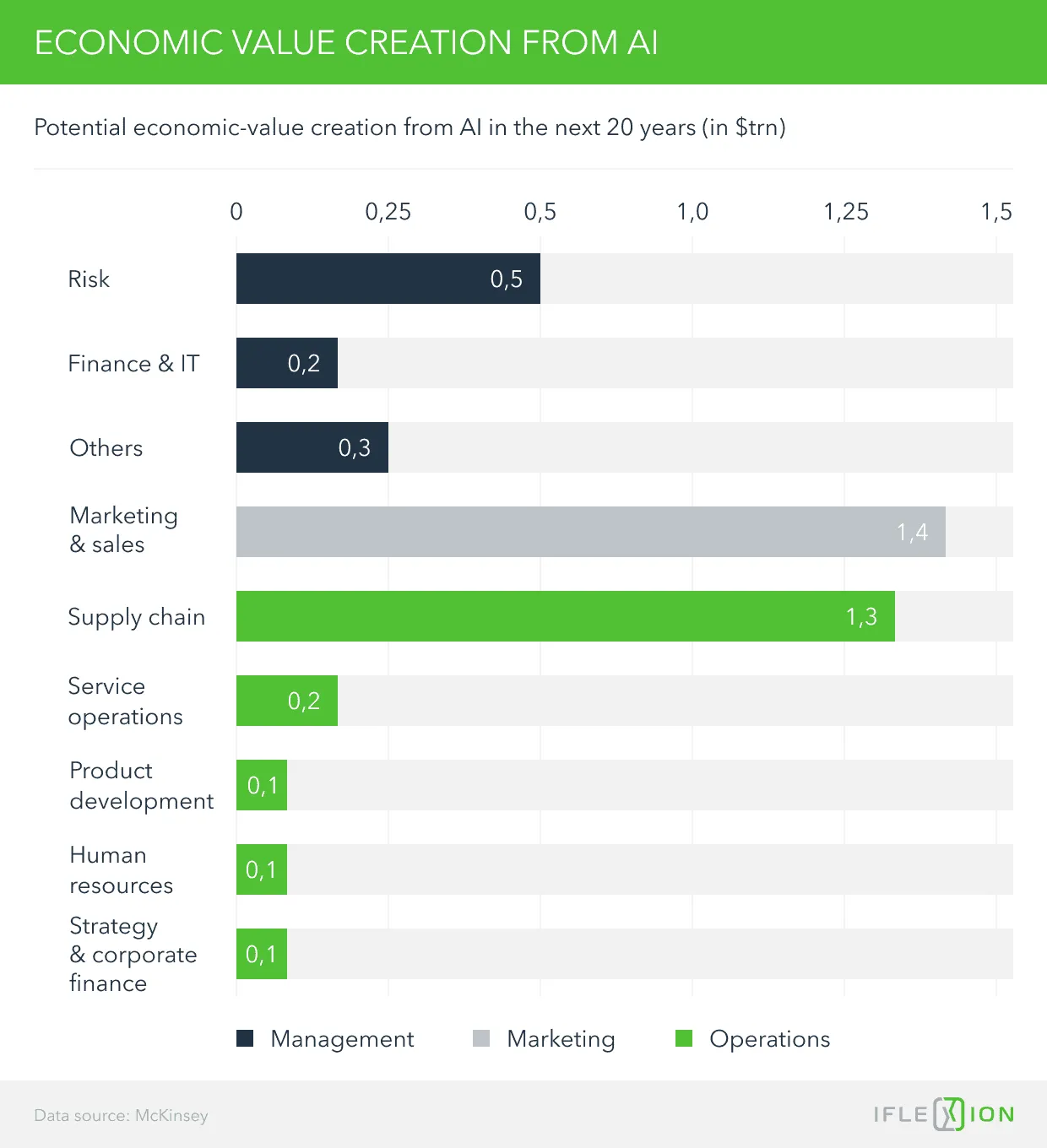
And while many manufacturers learned to minimize the damage of bad supply chain management (SCM), they can’t get away from the fact that manufacturing is becoming more complex. Eventually, even the most stable SCM system will succumb to rapid change if the issues are not addressed. Just look at the automotive industry. Cars today have more sensors, systems, and gadgets installed than ever before. It’s obvious that legacy supply management approaches can’t handle this variety.
That’s why Gartner placed AI as one of the biggest disruptors in supply management for 2018. Let’s talk about some of the more specific use cases.
Demand Forecasting
This one is the holy grail of supply chain management for any industry, but manufacturing especially benefits from its applications.
There are tons of existing legacy systems that can build demand predictions. But very few of them can truly be compared to the efficiencies offered by AI-augmented systems.
According to Aera Technology, a machine learning model is typically more accurate than standard forecasting tools by at least 10%. A survey of supply chain professionals by the Institute of Business Forecasting mentions that 70% of the respondents consider AI/machine learning technologies to be the future of demand analytics.
Automated Procurement
There are various venues for improvements here by applying AI in manufacturing. We’ll talk about just a couple of them.
- If you run a large manufacturing process, it’s likely that you have a procurement portal for suppliers. Chatbots can be used to automate some of the basic communications and help them apply for contracts.
- Identifying an optimal price equilibrium for contracts based on previous purchases and supplier history.
- Detecting supplier fraud risks based on procurement order details and supplier history data.
And this is just the tip of the iceberg. That’s why, according to Deloitte, 88% of procurement professionals predict that automation will highly impact their trade within five years. But let’s get back to the more production-focused applications of AI that are rising in popularity.
Yield Optimization
One of the most important production KPIs is yield. A variety of factors affects yield, but apart from reflecting productivity, yield reflects waste, as low yield comes hand in hand with faulty products or parts that have to be scrapped. According to Intel, a staggering 70% of materials can be wasted during a traditional manufacturing process.

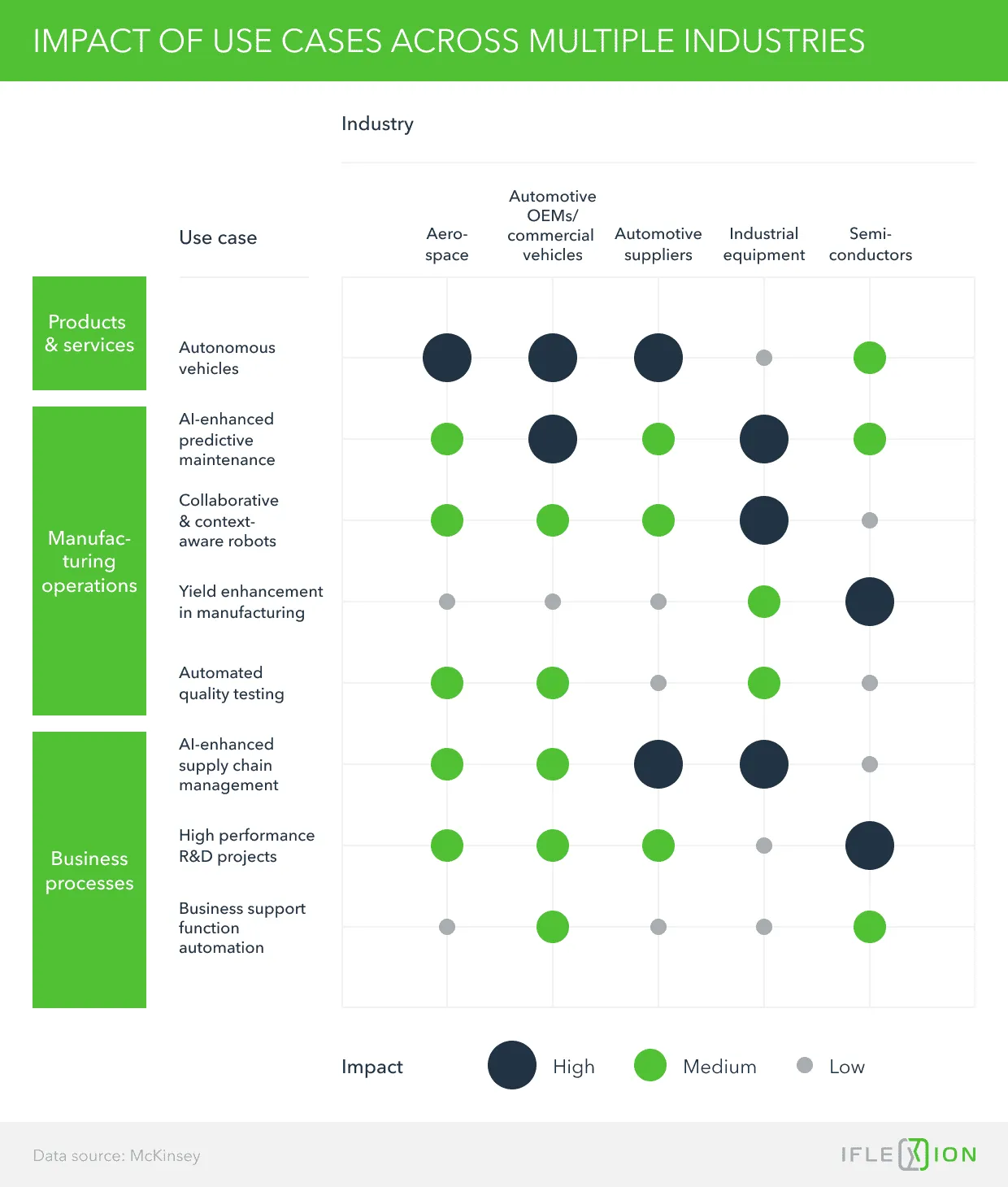
AI is the perfect solution to this problem, combining data from all of the involved subprocesses and production machinery. For example, automated AI systems in semiconductor manufacturing can reduce yield detraction by up to 30% (McKinsey).
Implement intelligent control and analytics into your project
Quality Assurance
These processes are an important part of the manufacturing process, which also has direct business ramifications. Poor quality of production may cost manufacturers their reputation and profits. Everybody remembers Samsung’s Note 7 fiasco. That’s why Samsung essentially doubled their battery QA efforts to eliminate similar dangerous situations in the future.
Visual inspections are a huge chunk of modern QA, and AI has made some significant advances in this domain. We recently discussed how AI is used for facial recognition. The same very basic principle applies here, but in this case machine learning algorithms learn to recognize product faults based on visual sensor data throughout the production and QA inspection processes.
But it’s not your simple facial data taken from a photo. We’re talking about infrared, X-ray, and other types of cameras that might be involved in the manufacturing process. According to the same report by McKinsey cited above, AI-based image detection can mean a 50% productivity improvement for fault detection processes and QA in general.
Facility Management
Warehousing spaces, production facilities, and administrative buildings are resource guzzlers demanding electricity, air conditioning, staff, etc. These costs can be optimized to improve energy efficiency and safety.
That’s exactly what Google is doing with their Deepmind AI, which now rules their datacenter’s cooling system. The AI periodically takes a snapshot of thousands of sensors across the datacenter, analyzes the data, and decides how to cool the environment. The result? A whopping 30% in energy savings. For manufacturers, this could mean millions of dollars in savings each year.

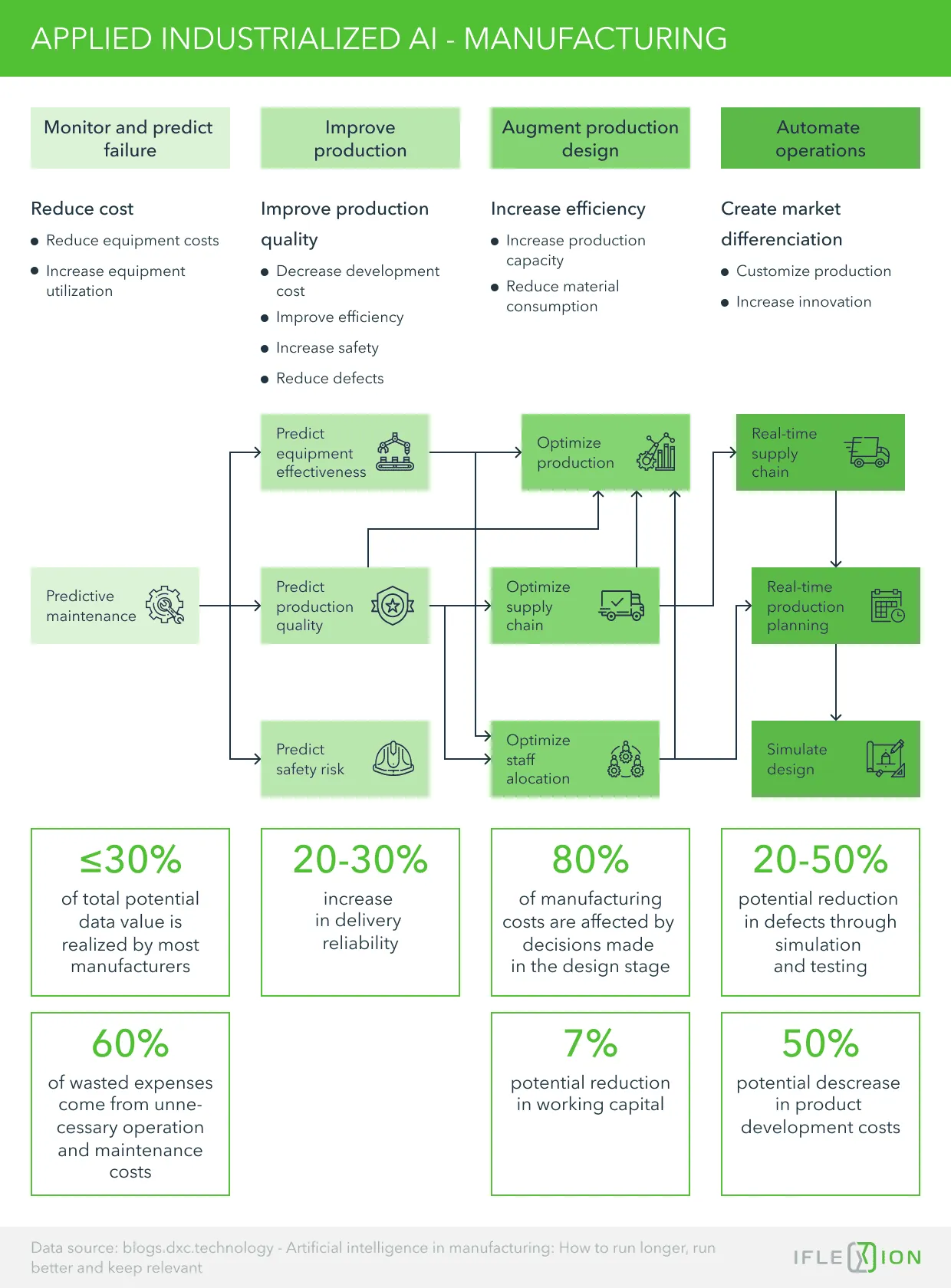
There are other use cases, like optimizing the utilization of your warehouse equipment. For example, AI can be used to predict the optimal number of forklifts required on any given day, based on operator performance and the potential demand for their work. If you’re renting equipment from a subcontractor that charges by the unit this could be a great cost-saving opportunity.
Business Optimization
This is a rather broad category bringing together interconnected use cases.
Warranty management is a great application for AI in manufacturing. Machine learning can be used to parse the return authorization text and warranty repair requests to identify potentially fraudulent claims. This data can also be used to predict the financial impact of each warranty repair request so manufacturers could prioritize more costly requests.
Staffing and talent management are other areas of significant impact. According to Deloitte, around a quarter of all people working in human capital (HR, etc.) already use AI to extend their capabilities. From staff allocation to safety risk predictions, AI can be used to optimize the efficiency of your workforce using the same methods and tools described above. For example, tools like Textio and MyInterview can help your HR department improve employee acquisition.
Post Scriptum
These use cases of AI in manufacturing are just a minuscule portion of the true potential for this industry. There are hundreds of use cases in administrative management, planning, sales, R&D, production, and aftercare. Manufacturers need to realize that while they weigh in on the benefits of AI development, their competitors are already implementing it. Technologies like automated machine learning only increase the velocity of the process by enabling more AI projects in much shorter timeframes.
Even if the initial impact of each specific use case is marginal, all of them add up in the long run, creating an immense competitive advantage through productivity and quality gains. Take a look around your specific manufacturing niche. Research your top competitor. It’s likely that they already have an AI project in the making or even in production. If they have a data scientist on board, the previous sentence is probably 100% true. So what are you waiting for?
At Iflexion, we understand the complexity and multidimensionality of manufacturing. We know where to look for opportunities and how to make sure that your AI project delivers value to production.
Bring intelligent automation and forecasting to your production line